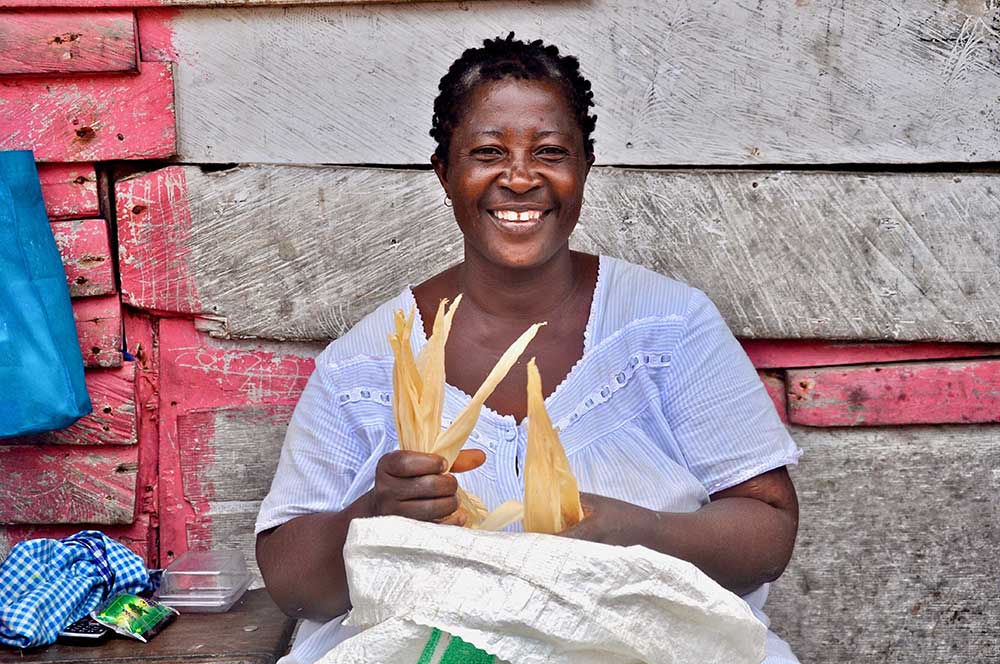
Assimila is working with Farmerline, University College London and CABI-Africa to develop an operational system for forecasting maize yields in Ghana using a crop model, coupled with Earth observations and weather data. Information will be provided down to the detail of individual farms – without the need for expensive and time-consuming ground surveys of crop growth. The forecasts are designed to be used by all those involved in the maize supply chain including farmers, maize traders and Government agricultural advisors.
Ian Jory, who is leading the project at Assimila says: “This work provides a perfect example of what Assimila does and how we operate. We are developing a practical, operational maize yield forecasting system – and we’re doing that using the combined expertise of Assimila and our partners in the science, data, information technology and markets. “
“This forecasting system simply wouldn’t be possible without Earth observation data to provide the up-to-date, field level monitoring of crop growth. But getting that information from Earth observation data is complex and therefore needs the expertise that Assimila has.”
Maize is a staple crop in Ghana, making up over 50% of the country’s cereal production and providing an important source of food. It also provides livestock and poultry feed and is used for a range of non-food products, such as matchsticks and biofuel.
Our partner Farmerline is an agriculture advisory business operating in Ghana. They have in-depth, first-hand experience of agriculture in Ghana and have been invaluable in helping us understand Ghanaian maize production and the information that different people need.
According to Amos Wussah, who leads the project at Farmerline “Smart farming decisions need information and that’s exactly what this forecasting system provides – based on cutting edge Earth observation technology combined with farm polygon on the Mergdata platform. Working with Assimila brings the vital expertise we need to unlock the opportunities of satellite data for agriculture – it’s proving to be a hugely productive partnership.”
The crop is grown mainly by smallholder farmers – who contribute around 90% of the country’s food production. Crops in Ghana are rain-fed and are extremely vulnerable to changing weather patterns. Production has been growing, but average yield remains well below potential. In fact, the yield in Ghana’s maize farms is one of the lowest in the world.
Smallholder farmers are just one part of the supply chain – traders, processors, sellers of agriculture fertilisers and seeds, researchers, finance and insurance providers are all part of the process. The Ghanaian Government is a major stakeholder, with programmes to modernise agriculture and a network of agricultural extension workers.
Trading of domestic maize depends mainly on a network of aggregators linked by personal and ethnic ties, as well as ‘market queens’ who trade at local and regional markets. Agribusiness/traders, also employ networks of agents buying at the farm level. These aggregators have to plan and organise logistics such as picking the maize crop, shelling, transportation and storage.
For all these actors in the maize supply chain, advanced knowledge of the season’s likely maize yields, could provide invaluable information. It might give a farmer increased bargaining power in negotiations over price, help plan logistics and reduce wastage, or guide national government to prepare for a potential maize shortage.
CABI-Africa, a partner in the project, is the African arm of CABI which provides information and expertise to solve problems in agriculture and the environment. CABI-Africa focussed on monitoring and evaluating the project. University College London is also involved, with whom Assimila have worked in the past on developing and applying data assimilation approaches to vegetation modelling.
How does our yield forecasting system work?
Maize in Ghana has two growing seasons each year: to coincide with the rainy seasons in spring and autumn. At the beginning of each growing season, a yield outlook can be provided at a regional level – giving an indication of how the harvest might compare with past years. More detailed forecasts can be provided, at more local scales, as the growing season progresses, with a yield estimate at harvest time at the farm level. Field/farm level information can be aggregated to give a broader, regional picture.
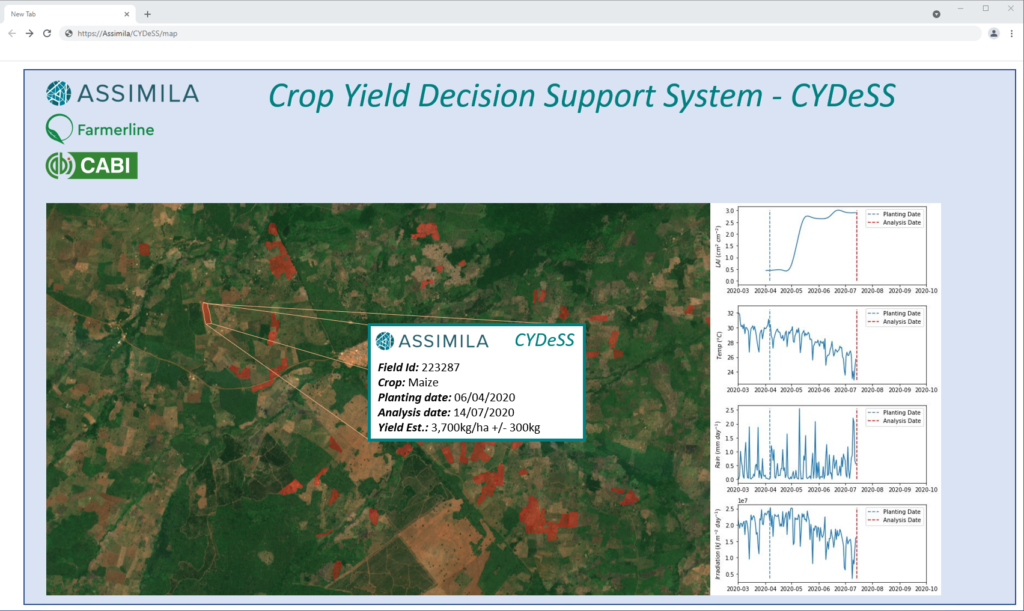
While crop forecasting often relies on statistical relationships, the Assimila approach is very much physically based. It uses the mechanistic World Food Studies (WOFOST) crop model coupled with Earth observations and weather data. By mechanistic, we mean that the model is simulating how the crop grows. Sunlight, rainfall and temperature form inputs and WOFOST then models how these resources are used by the crop to produce roots, stems, leaves and most importantly the maize cobs themselves. The WOFOST crop growth model is driven by current and forecast weather conditions to predict maize growth through the season, including forecasts of yield; it provides a suite (or ensemble) of possible outcomes.
Earth observation data are used to monitor the growth and health of the maize crop in near real-time (with a lag of 1 to 2 weeks). This Earth observation data is then used to fine tune the outcomes from the WOFOST crop model ensemble. The Earth observation data provide essential details of how the crop is developing on the ground in individual farms. For example, crop damage, from pests and disease or a localised storm, could be visible in the leaf area index signal derived from the satellite data.
The operational set-up would use Earth observations at key points in the growing season to check how well the maize crop is growing and to provide increasingly detailed forecasts of the final yield in tonnes per hectare.
The potential impacts of climate change could also be investigated using the system with scenarios of future yield at the regional scale, taking account of potential increases in temperature and variations in rainfall. Looking backwards in time, archives of Earth observations and weather data provide information on past yields at the farm scale, offering a consistent timeseries for benchmarking, trend analysis, and risk assessment.
These forecasting techniques have been tested previously in the UK and China on winter wheat and maize with excellent results. Field-level yields were forecast to within around 10% of what was observed.
Innovative use of Earth observation data
Assimila are using a multi-sensor satellite approach in this project.
The growth and health of the maize crop, and other vegetation, can be monitored from a range of satellite sensors. Such Earth observation data can give a view of crop conditions at farm level, down to a resolution of around 10 m and with a time lag of 1 to 2 weeks. Earth observation provides the only viable way to get such detail and wide spatial coverage.
Vegetation biophysical parameters such as leaf are index (LAI) are derived from satellite data using data assimilation techniques, involving a radiative transfer model to link land surface characteristics to the radiance measured from satellites. Leaf area index is a measure of the extent of a plant’s leaves and provides a good indication of plant growth. Optical data from the ESA Sentinel-2 satellite were a primary source in this work.
MODIS and Sentinel 3 Ocean and Land Colour Instrument, combined with forecasts of atmospheric aerosols, are used to correct the Sentinel 2 optical data which can be distorted by aerosol effects.
Novel techniques have been developed to use SAR (Synthetic Aperture Radar) data from Sentinel 1. SAR data have the huge advantage that they can ‘see’ the surface day and night and can see through clouds. Clouds, of course, are persistent over Ghana during the rainy seasons of interest, and they obscure the optical images from Sentinel 2. SAR backscatter data can give a picture of surface roughness and it is possible to see the gradual emergence of the maize crop as it grows. With complex processing, this SAR data can provide biophysical crop parameters, such as LAI, to supplement the parameters derived from Sentinel 2 optical data.
What comes next?
Currently the system is in a development and testing phase, which includes plans for the design and implementation of an operational service. Operationally the service could provide on-demand and routine crop yield information across the whole crop supply chain with products tailored to the needs and accessibility of the users. Furthermore, this service will be implemented as a sustainable business – modelled on the user requirements established during extensive stakeholder engagement.
The techniques employed have great potential for expansion to other crops and areas of the world.
Project and funding details
Project title: Crop Yield Decision Support – Enhancing Maize production resilience to climate change through advanced crop modelling and yield forecasting
The project was funded under the UK Space Agency (UKSA) International Partnership Programme (IPP).
UKSA IPP projects are designed to meet UN Sustainable Development Goals, such as support for precision agriculture, early warning systems for disaster prediction, maritime safety, and disease forecasting. All IPP projects are match-funded by consortium members and international partners to ensure maximum value for money.